Artificial Intelligence and Machine Learning Lab
Office and important requests:
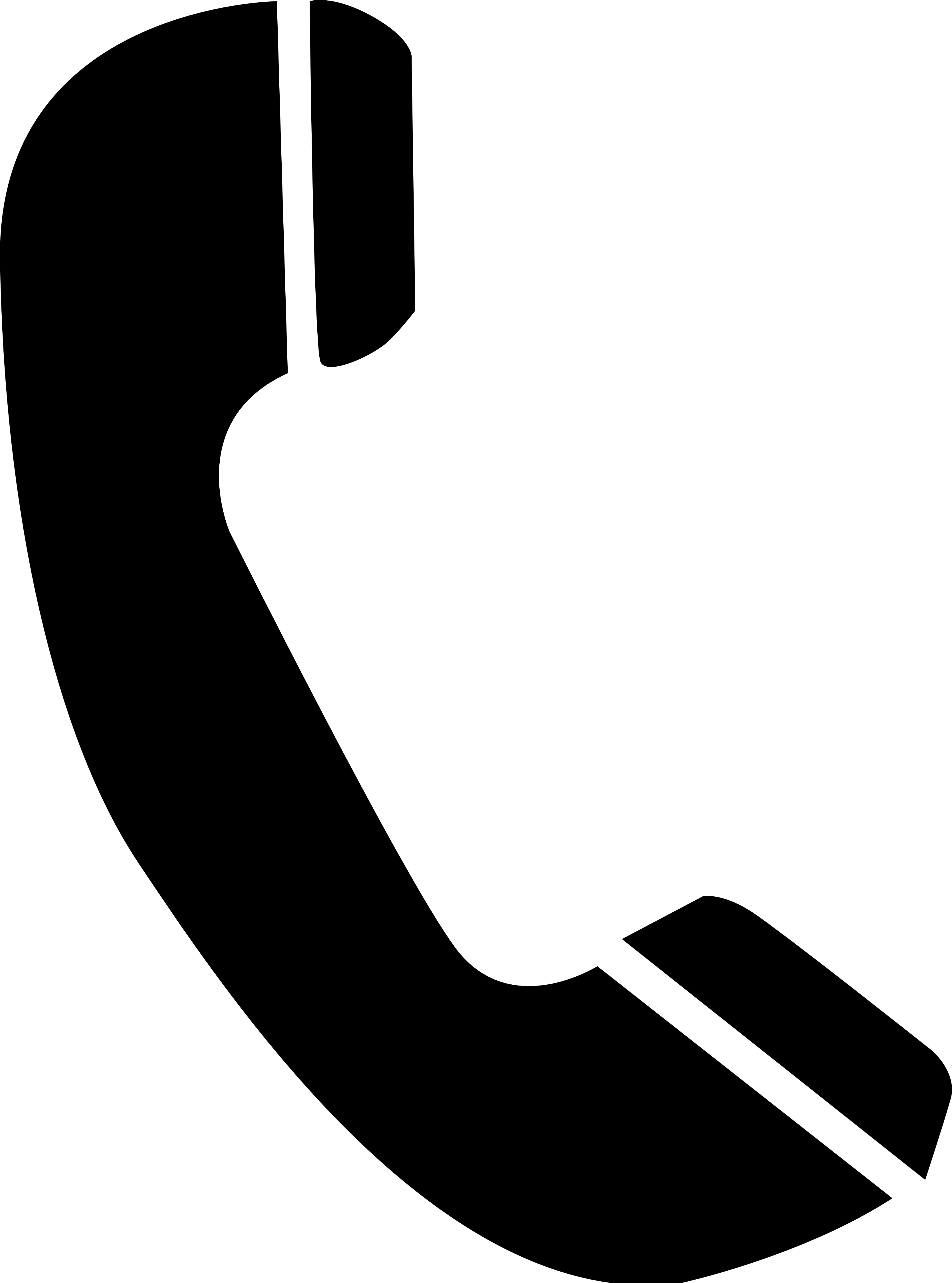
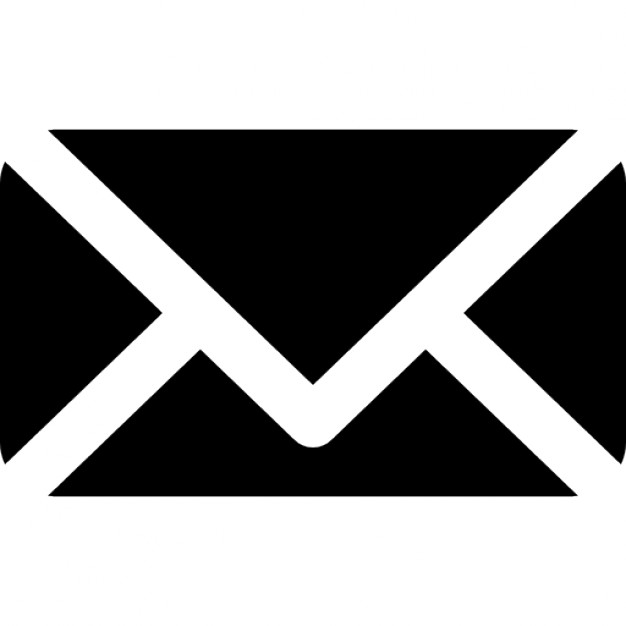
Meetings by appointment, general consultation: thursdays, 13:30-14:30 o'clock
Open Thesis Topics: please refer to our Thesis Proposal Instructions and follow the procedure listed there. Note: thesis supervision is only available for students of the TU Darmstadt.
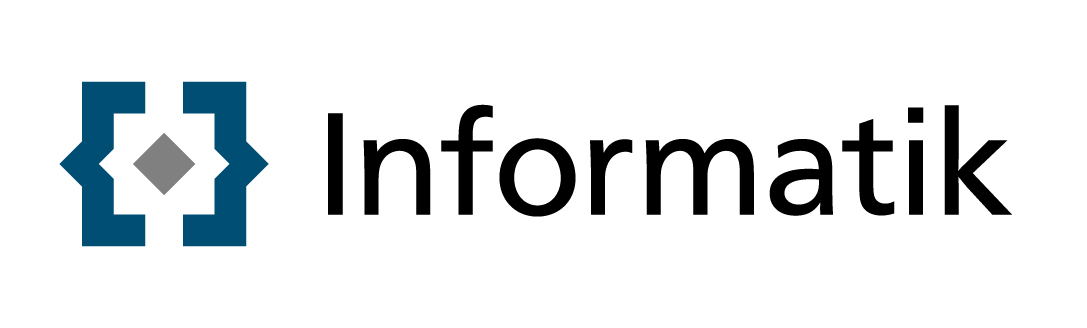
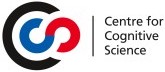
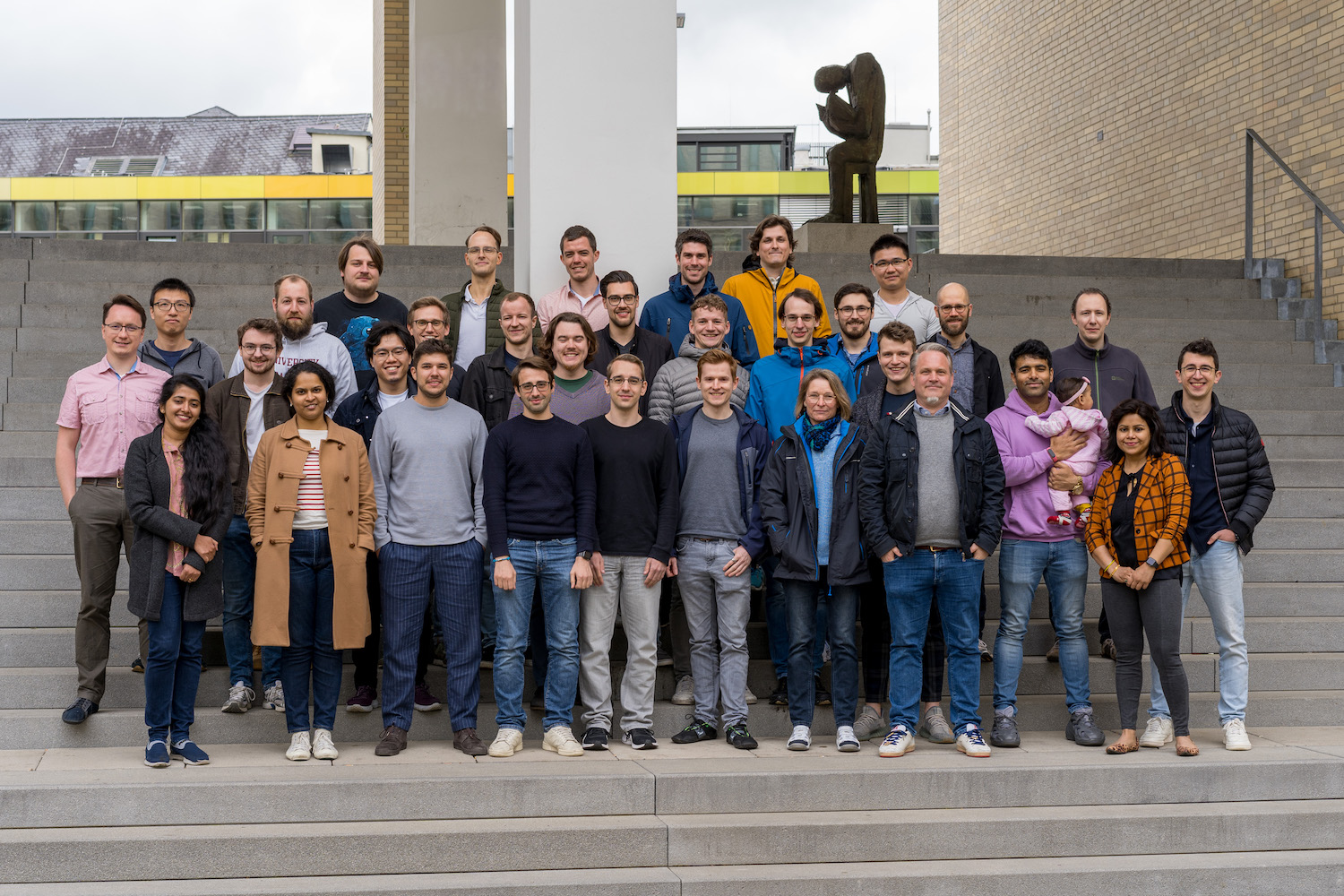
AIML Mission. The Artificial Intelligence and Machine Learning lab would like to make computers learn so much about the world, so rapidly and flexibly, as humans. This poses many deep and fascinating scientific problems: How can computers learn with less help from us and data? How can computers reason about and learn with complex data such as graphs and uncertain databases? How can pre-existing knowledge be exploited? How can computers decide autonomously which representation is best for the data at hand? Can learned results be physically plausible or be made understandable by us? How can computers learn together with us in the loop? To this end, we develop novel machine learning (ML) and artificial intelligence (AI) methods, i.e., novel computational methods that contain and combine for example search, logical and probabilistic techniques as well as (deep) (un)supervised and reinforcement learning methods.
AIML News.
March 2020: | Our work on using deep learning for playing the chess variant of Crazyhouse accepted at Frontiers in ML an AI. Congrats Johannes! |
March 2020: | We co-organized the Spring Symposium 2020 of the Fachgruppe Database Systems (FG DB). Thanks to Carsten for the co-organization and to all participants! |
Feb. 2020: | Our work on using SPNs for AQP within databases accepted at VLDB. Congrats! |
Feb. 2020: | Our work on estimating the importance of relational features accepted at ISMIS. Congrats Matej! |
Feb. 2020: | Looking for AI experts in academia with a proven technical competency in your country? We introduced together with Andreas Hotho from U. Wuerzburg AI-Rankings. Check it out! |
Feb. 2020: | Our Dagstuhl Seminar on "Software Engineering for AI-ML-based Systems" (SE4ML) was a lot of fun and very informative. Thanks to Miryung, Guy, and Thomas for the great coorganization! |
Jan. 2020: | Kristian appointed to the Research Council of the University of Hamburg and to the working group "Handling of Research Data" of the Wissenschaftliche Kommission Niedersachsen. |
Jan. 2020: | Extended abstract on Sum-Product Logic accepted at ProbProg 2020. Congrats! |
Jan. 2020: | Paper on private SPN inference accepted at ECAI 2020. Congrats Alejandro! |
Jan. 2020: | Our opinion on neural-symbolic models for argument mining accepted at Frontiers in Big Data. Congrats! |
Jan. 2020: | Kristian gave invited talk on Hybrid AI at ACM India Joint International Conference on Data Science & Management of Data (CODS-COMAD) 2020 in Hyderabad, India. |
Dec. 2019: | Two papers accepted at ICLR 2020, one on learning activation functions and one on video-based physics prediction. Congrats to everyone! |
Dec. 2019: | Kristian named ELLIS Faculty as part of the new ELLIS Unit IS•DA - Intelligent Systems at TU Darmstadt. |
Dec. 2019: | Kristian is founding board member of AI Frankfurt Rhine-Main, an AI network connecting companies, universities, research institutes and start-ups in the Rhine-Ruhr metropolitan region. |
Dec. 2019: | Talks on deep probabilistic models at the University of Waterloo and Borealis.AI, Canada. |
Nov. 2019: | Invited talk on the future of AI at the 50 Years Anniversary Conference of the University of Bielefeld. |
Nov. 2019: | Talk on "The Third Wave of AI" at the DFG Committee on Scientific Instrumentation and Information Technology in Berlin, Germany. |
Oct. 2019: | Tutorial on the Automatic Statistician at the Deutscher IT-Leiterkongress 2019 |
Oct. 2019: | ARTE series showcasing some recent developments in AI such as our moral choice machine. |
Oct. 2019: | We are now the Artificial Intelligence and Machine Learning Lab, stressing our strong research interests in reasoning and learning. |
Sept. 2019: | Kristian recevied the German AI Award (Deutscher KI Preis) 2019, which comes with 100K Euros. The award is under the patronage of the BILANZ magazin and supported by Airbus, the Apeiron Investment Group, the BMW Group, the Otto Group and the Maschmeyer Group. |
Sept. 2019: | Research stay of Karl Stelzner with Adam Kosiorek, University of Oxford, on sequential attend-infer-repeat models. |
Sept. 2019: | Kristian discussed moral machines, predictive policing, and AI at a panel of the Bad Homburg conference on "AI: How can we trust algorithms?". Thanks to the panel for the very interesting insights! |
Sept. 2019: | Kristian publically discussed with Hessen Minister for Higher Education, Research and the Arts Angela Dorn and Jessica Heesen from the University of Tübingen about AI and ethics. |
Sept. 2019: | Kristian talked about AI in a podcast featured by "Hessen schaft Wissen". |
Sept. 2019: | Our Dagstuhl Seminar on "Logic and Learning" was a lot of fun and very informative. Thanks to Phokion, Michael, and Daniel for the great coorganization! |
August 2019: | Our work on the moral choice machine got featured in muli-media online presentation. |
August 2019: | Our work on the moral choice machine got featured in the Frankfurter Rundschau |
August 2019: | Paper on explicit feature maps of graph kernels expected at DAMI. Congrats Niels and Chrisophter! |
July 2019: | Kristian joint the IJCAI-JAIR 2019 Best Paper Award Committee. |
June 2019: | Our paper on speeding up AIR received the Best Paper Award at the ICML 2019 Workshop on Tractable Graphical Models (TPM). Congrats Karl and Robert! |
June 2019: | Kristian elected as Fellow of the European Association for Artificial intelligence (EurAI) for exceptional contributions to the field of AI. |
June 2019: | Paper accepted at Remote Sensing on hyperspectral imaging at the ultraviolet range. Congrats Patrick! |
May 2019: | UAI paper accepted on deep probabilistic learning using random sum-product networks. Congrats Karl, Alejandro, Robert, Antonio and Martin! |
May 2019: | IJJR paper accepted on probabilistic movement grammars. Congrats Rudi! |
May 2019: | Kristian joint the KDD 2019 Best Paper Award Committee for the Applied Data Science Track. KDD is the premier interdisciplinary conference bringing together researchers and practitioners from data science, data mining, knowledge discovery, large-scale data analytics, and big data. |
May 2019: | Kristian joint the jury of the "Deutscher KI-Preis" of the German Magazin BILANZ in cooperation with Airbus, Presight Capital, the BMW group, McKinsey&Company and the Otto group. |
April 2019: | Accepted ICML paper on making the AIR model faster using SPNs. Congrats Karl and Robert. |
April 2019: | Karl shared his views on AI at the 6. Wiesbadener Science Pub. |
April 2019: | Krisitan joint the Expert Committee of the Association of German Engineers (VDI) for fundamentals of intelligent learning systems. |
April 2019: | Invited Talk at DFG conference on "Traceability and securing of results as essential challenges of research in the digital age", Berlin, spring 2019. |
April 2019: | Invited Talk at 3rd ETAPS Workshop on Learning in Verification (LiVe). |
April 2019: | Discussion with high school students on Blade Runner and Artificial Intelligence as part of the Schulkinowochen in Hesse. |
March 2019: | Consider to submit to our ICML workshop on Tractable Probabilistic Models (TPMs). Congrats Alejandro for coorganizing this. |
March 2019: | Keynote on AI at the Leibniz Convent on Artificial Intelligence. |
March 2019: | Our "Moral Choice Machine" featured on HR-fernsehen, the television station of Hessischer Rundfunk, starting in the video at around 13:15 min. |
March 2019: | Kristian was named Top 100 Influential Scholar 2018 for Artificial Intelligence by AMiner. The 2018 winners are the most cited scholars in the AAAI and IJCAI conferences between 2007 and 2017, which are identified as the top venues of Artificial Intelligence. |
Feb. 2019: | Invited talk on AI at the EFL Spring Conference on AI in the financial service industry. Thanks for organizing such a great event! |
Feb. 2019: | Full week AI/ML course for Bat the Bosch Innovation Campus in Renningen. Thanks for the great feeback! |
Jan. 2019: | Acquired a new RMU Network for Deep Continuous-Discrete Machine Learning (DeCoDeML). The goal is to make deep models more comprehensible or at least perceived in such a way that they can be related to human understanding. |
Dec. 2018: | Two papers accepted at AIES, the second ACM/AAAI conference on AI, ethics, and society. The one paper introduces the novel paradigm of Explanatory Interactive Machine Learning, the other one the Moral Choice Machine. Congrats guys! |
Dec. 2018: | Kristian joint CLAIRE's IAG ML, which should serve as a good mechanism for coordinating between CLAIRE and ELLIS. Exciting! ML and AI are two fellow travelers on the quest for intelligent behaviour in machines, and Darmstadt is supporting both ELLIS and CLAIRE. |
Nov. 2018: | The AI•DA-CLAIRE Symposium about the the beginnings, the present and the future of AI-research on the occasion of Wolfgang Bibel's 80th birthday was a great success. Thanks to all speakers for their wonderful talks! |
Oct. 2018: | Two AAAI 2019 papers accepted. Congrats guys! |
Oct. 2018: | Check out Kristian's interview within the AI Profiles column of AI Matters 4(3), 2018 |
Oct. 2018: | New project as part of the RMU "Initiavfonds Forschung" for setting up a collaboration with the Universty of Mainz on deep learning in mixed domains. Looking forward to our collarbotion Stefan! |
Oct. 2018: | Chancellor Dr. Merkel describes the TU Darmstadt as a "jewel in questions of AI with all its sub-areas". |
Oct. 2018: | Invited talk on "Towards the Democratization of Machine Learning using Probabilistic Programming" at the Inaugral Conference on Probabilistic Programming (ProbProg) 2018. |
Oct. 2018: | Invited talk on "Feeding the world with big data: machines uncover spectral characteristics and dynamics of stressed plants" at "Plants and Animals: Bridging the Gap in Breeding Research" 2018. |
Sept. 2018: | Invited talk on "The Automatic Data Scientist" at the Logic and Learning section of the Highlights of Logic, Games and Automata 2018 conference. |
Sept. 2018: | Our KI 2018 tutorial on "Statistical Relational AI" in Berlin was a great success. Thanks to Tanya and Ralf! |
August 2018: | TUDa is the #1 German and #2 European AI institution (based on the number of publication at top venues over the last 10 years in the combined areas of Artificial Intelligence, Computer Vision, Machine Learning, Natural Language Processing, and Robotics) according to csrankings.org. |
August 2018: | Our European Association for AI (EurAI) Advanced Course on AI (ACAI) "Statistical Relational AI" in Ferrara (Italy) was a great success. Great lecture materials and recordings! Thanks to all lecturers and the whole team for the great event! |
August 2018: | The German Workshop on Knowledge Discovery and Machine Learning (KDML) 2018 we organized in Mannheim (Germany) was a lot of fun. Thanks to the whole team for the great organization and support! |
August 2018: | The International Conference on Uncertainty in AI (UAI) 2018 we helped organizing in Monterey (USA) was a lot of fun. Thanks to the whole team for the great organization and support! |
July 2018: | Invited talk on "Systems AI" at IJCAI 2018 workshop on Learning and Reasoning Workshop (LR) |
July 2018: | Hosted interim meeting of our "Artificial Intelligence" Research Training Group of the German National Academic Scholarship Foundation at the TU Darmstadt. Thanks for all the exciting discussions! |
June 2018: | Two Dagstuhl seminars accepted: "Logic and Learning" and "Software Engineering for AI-ML-based Systems." Exciting! |
June 2018: | The call for the establishment of a confederation of laboratories for AI research in Europe (claire-ai.org) is out. We are a key supporter! |
June 2018: | Participated in BMBF expert panel on "Made in Germany: Self-learning systems as Advantage for Tomorrow" at CEBIT 2018. |
June 2018: | Three TPM@ICML2018 papers accepted. Congrats guys! |
June 2018: | Founding EiC of the new Frontiers in Big Data journal on "ML and AI". Check it out! |
May 2018: | Keynote on "Automatic Data Scientist" at Data Science Day Jena 2018. Thanks for inviting! |
April 2018: | One IJCAI-ECAI survey paper on "Systems AI" and learning-based programming accepted! Congrats Parisa! |
April 2018: | Joint the Technical Review Board of the Robert Bosch Centre for Data Science and AI at IIT Madras, India. This is an interdisciplinary research centre for AI set up at IIT Madras. Exciting! |
April 2018: | Two IJCAI-ECAI papers on symbolic-numerical and lifted inference accepted! Congrats to all! |
Feb. 2018: | Joint the working group "Technoligical Enablers and Data Science" of the Plattform Self-Learning Systems of the Federal Ministry for Education and Research (BMBF). Excited to help shaping the future of AI in Germany. |
Jan. 2018: | One SysML and one ICRA paper accepted! Congrats Rudolph and Alejandro! |
Dec. 2017: | Our NIPS 2017 tutorial and workshop went very well. Thanks for attending! |
Nov. 2017: | And the P5 Award 2017 goes to the Project Group 608 "Manipulation". Congratualtions guys! |
Nov. 2017: | Three AAAI papers about core sets, sum-product networks, resp. autoencoders accepted. Congrats guys! |
Oct. 2017: | Children's university lecture on "What is actually Artificial Intelligence?" at the comprehensive school Gänsewinkel in Schwerte, Germany. |
Oct. 2017: | Consider to submit to our NIPS 2017 Highlights workshop! |
Oct. 2017: | New DFG project on Argumentative Machine Learning (CAML) within the SPP RATIO. Looking forward to our collaboration, Matthias! |
Oct. 2017: | Invited talk on "Tractable Data Journalism" at the Berlin Machine Learning Meetup Group. |
Sept. 2017: | BIBM paper on predicting the number of Angioplasty procedures using Poisson Dependency Networks accepted. Congrats Shuo! |
Sept. 2017: | Invited talk on declarative machine learning systems and lifted quadratic programming at the KI 2017 Sister Conference Session. |
Sept. 2017: | Course on "Tractable Probabilistic Graphical Models" at the 4th International Summer School on Resource-aware Machine Learning. |
Sept. 2017: | Consider to join our EurAI sponsored ACAI Summer School on Statistical Relational AI in Ferrara, Italy, 2018. |
Sept. 2017: | Consider to submit to our AAAI 2018 workshop on Declarative Learning Based Programming (DeLBP). |
Sept. 2017: | First week of our "AI - Facts, Chances, Risks" Research Training Group of the German National Academic Scholarship Foundation. |
Sept. 2017: | Invited Talk on "Tractabel Data Journalism" at SciCAR. |
Sept. 2017: | Consider to join our NIPS 2017 tutorial Statistical Relational AI on how to combine relational and probabilistic AI. |
Aug. 2017: | ICDM paper on randomized high-dimensional Weisfeiler-Lehman graph kernels accepted. Congrats Christopher! |
Aug. 2017: | Organizing UAI 2017 in Sydney was a lot of work but also fun. Thanks to everyone making UAI 2017 such a great experience. |
Aug. 2017: | Our KDD 2017 workshop on "Data Science meets Journalism" (DS+J) went very well. Thanks to everyone! |
July 2017: | Our BLE project on Deep Learning for Plant Phenotyping finally started at the TU Darmstadt. Welcome Patrick! |
June 2017: | Invited talk at CPAIOR 2017 about declarative machine learning systems and lifted quadratic programming. |
May 2017: | Moved to TU Darmstadt. Thanks to TU Dortmund for wonderful years. |
April 2017: | Consider to submit to our KDD 2017 workshop on "Data Science meets Journalism" (DS+J) |
April 2017: | PhD course on how to combine relational and statistical AI at the University of Trento. Thanks Andrea for a wonderful stay. |
Jan. 2017: | Distinguished lecture on declarative ML systems at the University of Jena. |
Jan. 2017: | Three papers at AAAI 2017. Congratulations guys! |
Dec. 2016: | Invited talk on ML Systems at the NIPS 2016 Workshop on "Neurorobotics: A Chance for New Ideas, Algorithms and Approaches" |
Sept. 2016: | Invited talk at DS 2016 on mathemtical models of collective attention. |
Sept. 2016: | Invited talk at BeneLearn 2016 on declarative machine learning systems. |
People
Teaching
Publications
Funding
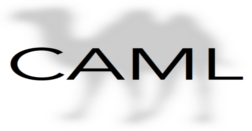
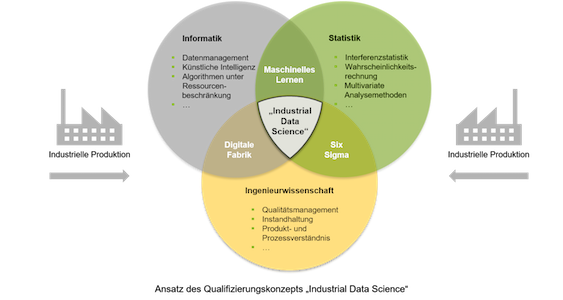

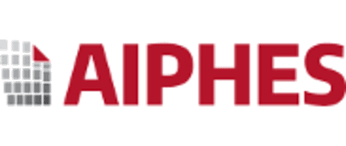
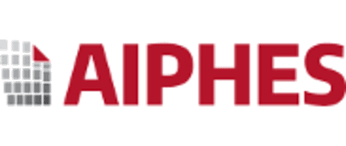
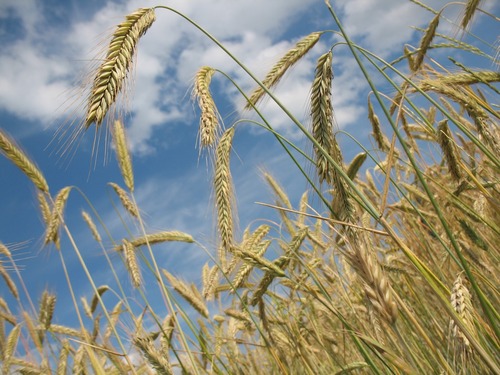
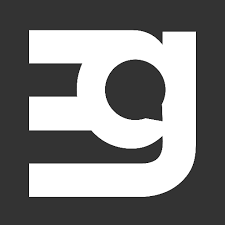
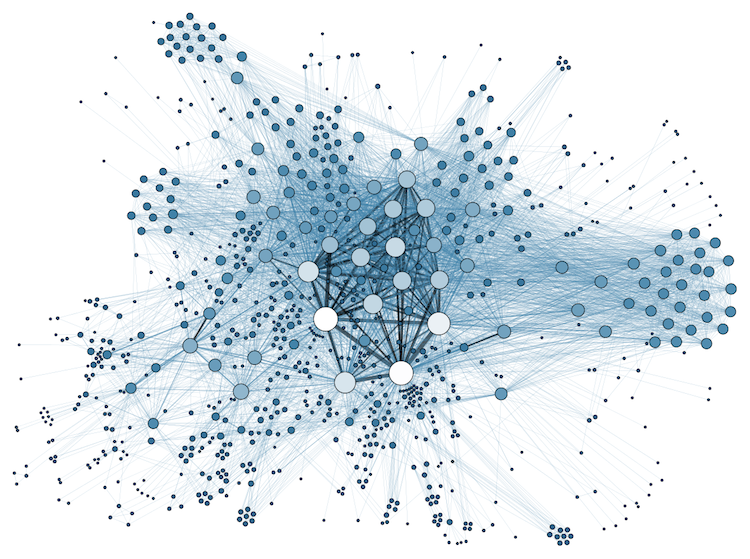
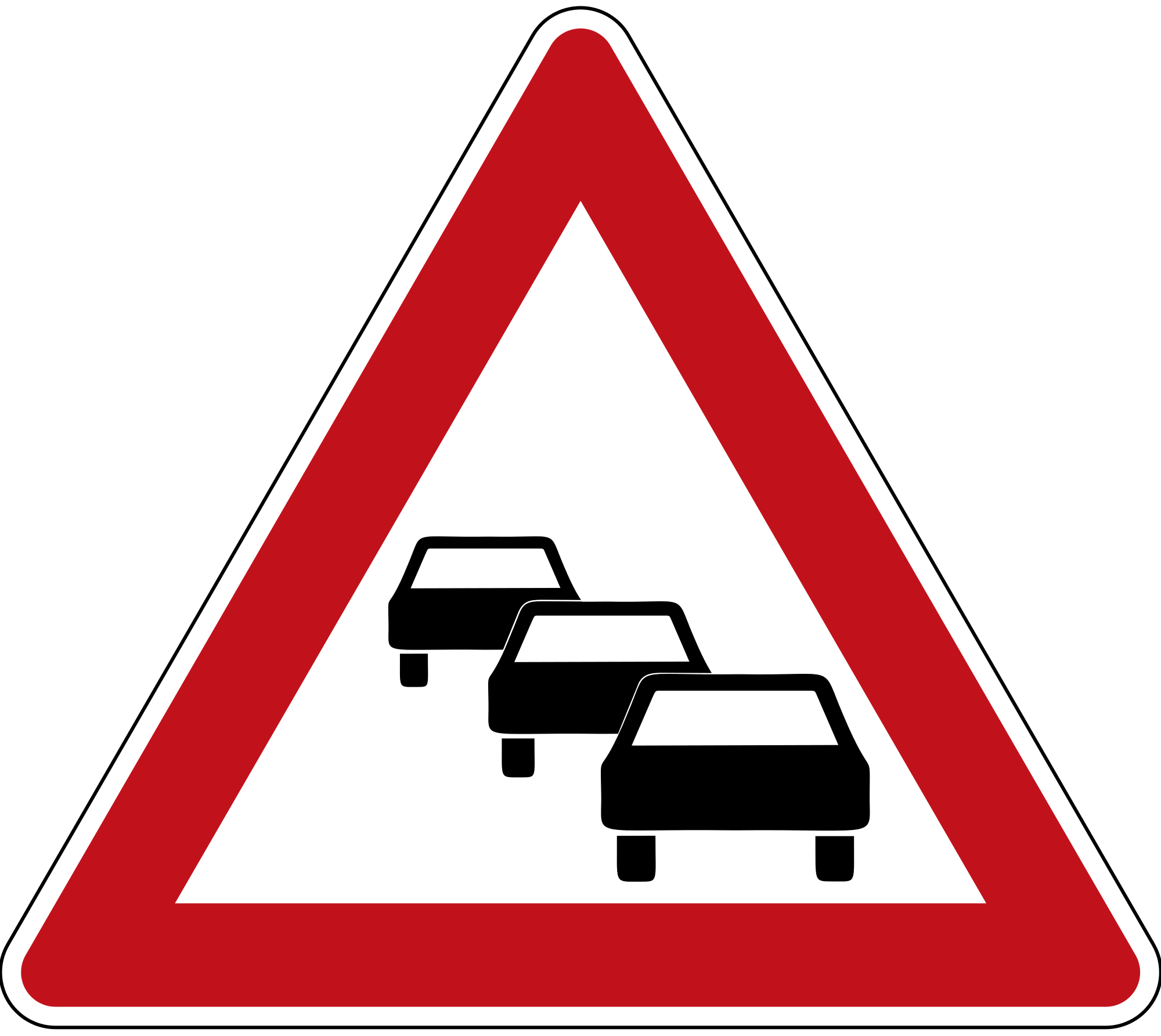
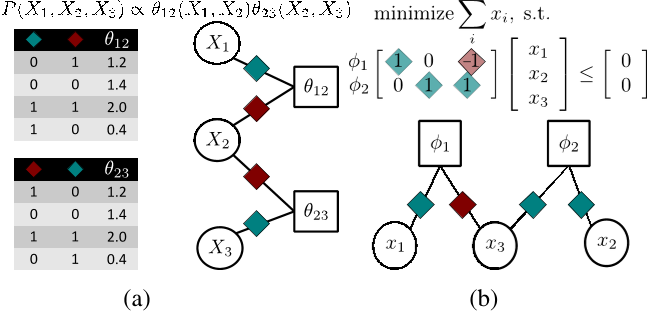
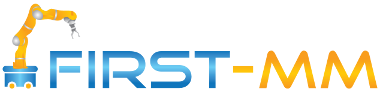
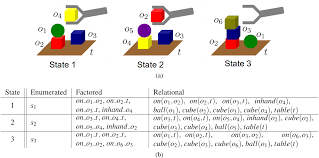
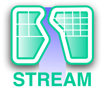
Most existing activity mining approaches indeed take uncertainties into account, but they do not consider the rich relations among people and objects that exist in the real world. STREAM’s goal was to develop formalisms, models, and algorithms for effective and robust statistical relational activity mining that enables one to develop socio-cognitive aware systems and to apply them on significant real-life applications. To this aim STREAM develop statistical relational learning methods using a Fraunhofer ATTRACT fellowship.
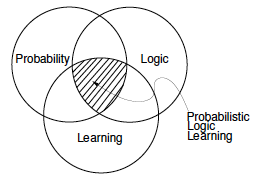